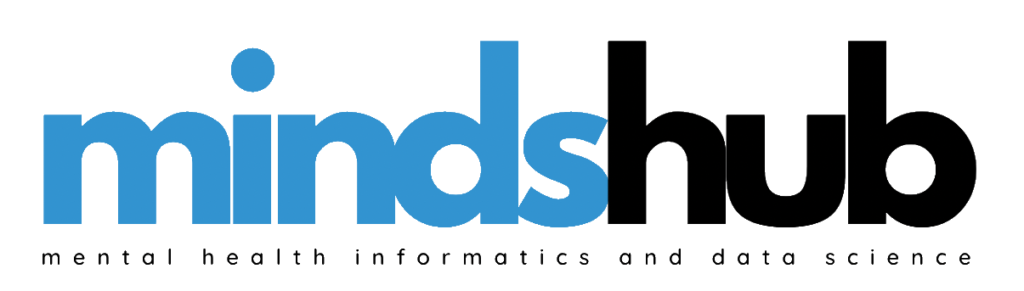
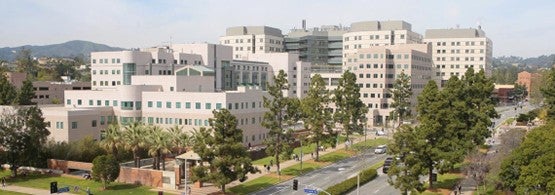
Electronic Health Record (EHR) Phenotyping
In adolescence
We are developing methods to use healthcare data to track and monitor emergency care for self-injurious thoughts and behaviors (SITB) among children and teens. Our work was featured at the 2023 American Medical Informatics Association Annual (AMIA) Summit and received the Clinical Research Informatics Distinguished Paper Award.
Our team found that using suicide-related diagnostic codes miss nearly one-third of SITB cases, while suicide-related chief complaints miss more than half. Detection accuracy vary based on the child’s gender and age, with lower sensitivity for male children and preteens compared to female children and adolescents. Certain types of SITB, like preparatory acts and attempts, are also more challenging to detect accurately.
To improve detection, we are developing and validating machine learning models using natural language processing of clinical notes, structured health record data, and gold standard classifications by experts. Early results suggest these models significantly improve sensitivity compared to those relying solely on suicide-related codes and chief complaints. Read our research paper published in JMIR Mental Health.
Support for this research comes from:
In early childhood
Suicide is the second leading cause of death in the U.S. among children 10 to 14 years old. Self-injurious thoughts and behaviors present differently with age and monitoring of suicide-related emergencies may be improved by accounting for ontogenetic variation in self-injurious thoughts and behaviors. In this study, we test the performance of current data mining methods in detecting suicide-related emergencies among elementary school-aged children (6-to-12 year olds). We then adapt a series of developmentally-informed machine learning models using natural language processing and structured medical record data with the goal of improving detection of suicidal thoughts and behaviors among children.
This study is supported by a research grant from the Thrasher Research Fund.
Childhood-onset mental illness is a national priority area, underscored by the rapid rise in clinical need. Discovery of children with mental health (MH) disorders using electronic health records (EHRs) is a challenging task due ontogenetic variation in illness phenotypes along the developmental continuum.
Current detection methods are often imperfectly extrapolated from adult phenotypes, rely on poorly sensitive rule-based algorithms (e.g., diagnostic codes) or deploy supervised approaches trained using resource-intensive manual chart review.
Our research applies advances in semi- and weak-supervised approaches to develop phenotypes of childhood onset mental illness using medical record data.
Support for this research comes from: